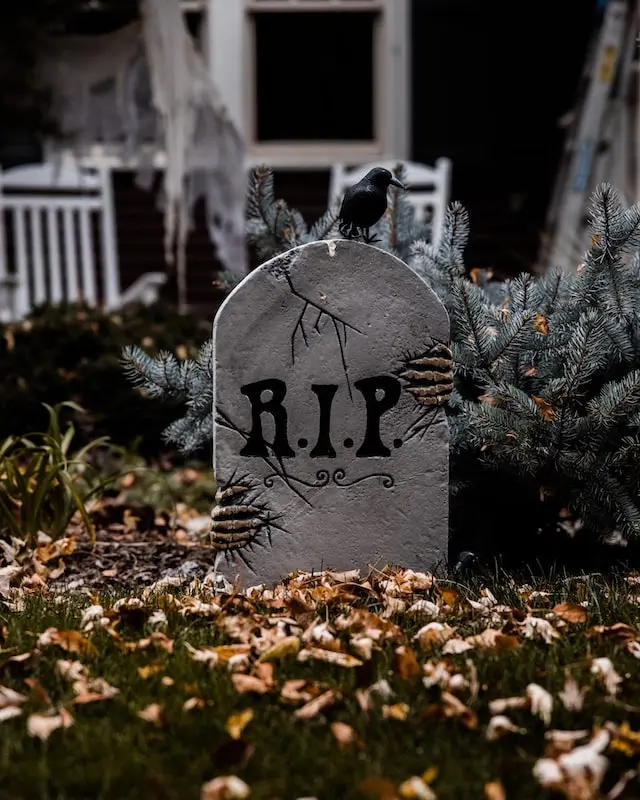
You may have come across a pretty popular article published early this month with the thesis that Big Data is Dead because:
-
Most people don’t have that much data.
The median data size among heavy BigQuery users was much less than 100GB. -
Most workloads need to process only a small amount of the total data.
Even when there is Big Data, most queries process only recent data (daily, weekly, monthly), and use aggregations of the older data. 90% of the workloads process 100MB of data. So the compute size grows disproportionately slower than the data storage size. -
The Big Data Frontier keeps receding.
One of the definitions of Big Data is “whatever doesn’t fit on a single machine.” A single machine can now process several orders of magnitude of data than what was possible 15 years back. What was Big Data back then is small data now. -
Data is a Liability.
Too much data, especially old data, may have serious legal and privacy repercussions.
So, unless you are in “Big Data 1%”, you are better off with data tools that are apt for “data at the size you actually have, not the size that people try to scare you into thinking that you might have someday.”
Nobody can quarrel with that thesis. However, the real issues are the points barely touched upon at the beginning and toward the end of the article:
-
Data Quality: “People have a hard time gaining actionable insights from their data has been blamed on its size,” and
-
Data Quantity: Another definition of Big Data is “when the cost of keeping data around is less than the cost of figuring out what to throw away.”
In other words, data quality is the real problem causing data lakes to turn into data swamps, where only a small island of truth is trustworthy.
So, while that thesis has technical merit, Big Data will live as long as humans have this foolish greed that let’s collect whatever data we can collect and we will figure out later what to do with it. Combine it with fatal laziness and being sloppy in recording exactly what the collected data is and how it was collected, and I bet the revenues of Big Data scaremongers will continue to grow.
Big Data’s death has been predicted earlier too
-
Big Data Is Dead: Long Live Smart Data, Forbes, Mar 2021
-
The Death of Big Data and the Emergence of the Multi-Cloud Era, KDnuggets, July 2019
Technically, Big Data may never die if you go by its characterization through 3 Vs: volume, velocity, and variety. And frankly, it doesn’t matter! You will find a lot of people claiming that they are sitting on a data goldmine but in reality it data garbage pile.
Another perverse politics of using data (and analytics) only as a rubber stamp for senior management’s prior beliefs as pointed out in the comments on the article at Hacker News:
I used to joke that Data Scientists exist not to uncover insights or provide analysis, but merely to provide factoids that confirm senior management’s prior beliefs.
I did several experiments, and noticed that whenever I produced analysis that was in line with what management expected — my analysis was praised and widely disseminated. Nobody would even question data completeness, quality, whatever. They would pick some flashy metric like a percentage and run around with it.
Whenever my analysis contradicted — there was so much scrutiny in numbers, data quality, etc, and even after answering all questions and concerns — analysis would be tossed away as non-actionable/useless/etc.
if you want to succeed as a Data Scientist and be praised by management — you got to provide data analysis that supports managements ideas (however wrong or ineffective they might be).
Data Scientist’s job is to launder management’s intuition using quantitative methods :)
The ordeals that data scientists, analysts, and engineers face are not new. There was an insightful piece 3 years back by a disillusioned data scientist: Data Science: Reality Doesn’t Meet Expectations. It highlighted 7 issues plaguing the industry (and the situation has not changed much since then):
-
People don’t know what “data science” does.
-
Data science leadership is sorely lacking.
-
Data science can’t always be built to specs.
-
You’re likely the only “data person.”
-
Your impact is tough to measure — data doesn’t always translate to value.
-
Data & infrastructure have serious quality problems.
-
Data work can be profoundly unethical. Moral courage is required.
Hacker News commentary on that article was interesting too. For example, one comment listed 4 common characteristics of successful data science projects:
A reasonably solid understanding of what the data could and couldn’t do. What can we actually expect our data to achieve? What does it do well? What does it do poorly? Will we need to add other data sets? Propagate new data? How will we get or generate that data?
The business case or user problem was understood up front. In our most successful project, we saw users continuously miscategorized items on input and built a model to make recommendations. It greatly improved the efficacy of our ingested user data.
Break it into small chunks and wins. Promising a mega-model that will do all the things is never a good way to deliver aspirational data goals. Little model wins were celebrated regularly and we found homes and utility for those wins in our codebase along the way.
Make is accessible to other members of the company. We always ensure our models have an API that can be accessed by any other services in our ecosystem, so other feature teams can tap into data science work. There’s a big difference between “I can run this model on my computer, let me output the results” and “this model can be called anywhere at any time.”
In summary, who cares if Big Data is dead! What really matters is the quality of the data, the data quotient of the organization, and the motives behind using data analytics.